The e-commerce sector, particularly for large and medium-sized retailers, faces a unique set of challenges in today’s digital marketplace.
These businesses have to figure out how to manage huge product catalogs, make customer experiences personal at scale, and boost conversion rates in a competitive market. They also have to deal with rising customer acquisition costs and the need to reduce return rates while staying profitable.
It turns out that Large Language Models (LLMs) have become a powerful tool to address most of these challenges.
While many are familiar with LLMs in the context of chatbots or basic product recommendations, their potential extends far beyond these applications.
This article explores five non-obvious yet highly effective ways to leverage LLMs in e-commerce operations, offering solutions to some of the most pressing issues online retailers face:
1. Intelligent Review Summarizer
Consider the potential of an LLM-powered User Review Summarizer and Responder. Such a system could go beyond basic sentiment analysis by extracting nuanced themes from customer reviews and identifying specific product strengths and weaknesses.
In theory, if multiple users praise a particular feature of a product, the system could automatically highlight this in the product description. This level of detail would allow for dynamic product information updates based on real customer experiences.
Moreover, this technology could generate tailored responses to reviews, maintaining a consistent brand voice while addressing individual concerns.
The impact of such a system, if implemented, could be significant.
Research indicates that 74% of consumers rely on ratings and reviews as a primary method for learning about products they haven’t purchased before. By effectively managing and leveraging this customer feedback, businesses could potentially influence potential buyers’ decisions and tap into a crucial source of information for new customers.
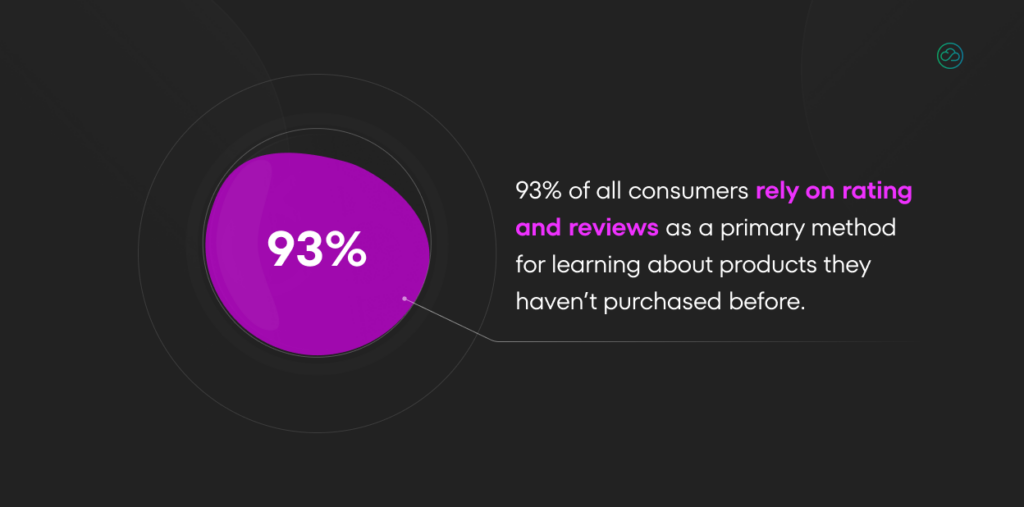
2. Natural Language Product Filtering
Product discovery, especially in categories with complex specifications, can be a major pain point for online shoppers. Traditional filter systems often overwhelm customers with too many options and technical jargon.
Imagine an LLM-powered “Filtering 2.0” system that allows customers to describe their needs conversationally, much like they would to a knowledgeable salesperson. For example, a customer shopping for a laptop could potentially input: “I need a lightweight laptop for video editing, with at least 16GB of RAM and good battery life.” The system would then translate these preferences into appropriate filters, presenting the most relevant products.
The potential impact of such a system is substantial. Recent research reveals a critical issue in e-commerce search functionality: 61% of all sites perform below an acceptable search performance level that aligns with users’ actual search behavior and expectations. Even more alarmingly, 15% of sites have what can be described as “broken” search query type performance.
These statistics underscore the urgent need for improved search and filtering systems in e-commerce. By implementing an LLM-powered natural language filtering system, businesses can potentially address these shortcomings, significantly enhancing the user experience and improving product discovery efficiency.
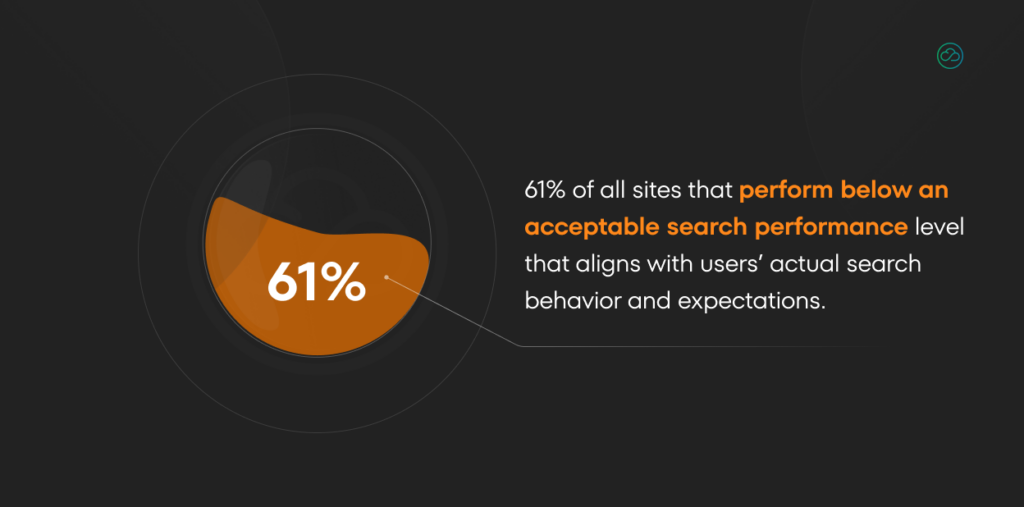
3. Dynamic Product Comparison
This tool could offer a more dynamic and personalized solution to product comparison. This theoretical LLM-based comparison engine would engage in a dialogue with customers, highlighting relevant differences based on their unique needs and use cases.
For example, when comparing digital cameras, the system could explain: “Given your interest in wildlife photography, Camera A’s superior autofocus system and higher frame rate would be more beneficial than Camera B’s slightly higher resolution.”
This level of personalized comparison goes beyond traditional side-by-side spec sheets, providing context and relevance to each customer’s specific situation.
The system could also adapt its language and depth of explanation based on the customer’s level of expertise. For a novice, it might focus on basic features and user-friendly aspects, while for an expert, it could delve into more technical details and advanced functionalities.
Furthermore, this dynamic comparison engine could potentially integrate real-world usage scenarios and user-generated content. For instance, it could pull relevant information from customer reviews or user forums to provide practical insights into how the products perform in real-life situations.
The importance of clear product information cannot be overstated. A study by the Nielsen Norman Group found that 20% of purchase failures are directly related to missing or unclear product information. By providing contextual, user-specific comparisons, e-commerce businesses can potentially increase conversion rates and reduce post-purchase dissatisfaction.
4. Contextual Product Expert System
A hypothetical Contextual Product Expert System, powered by LLMs, could offer a scalable solution to providing expert-level advice for complex product categories. This AI advisor would assess the customer’s level of expertise and use case, then provide tailored advice, replicating the experience of consulting with a knowledgeable salesperson.
The system could explain technical features in appropriate terms, suggest complementary products, and offer post-purchase support. For instance, a customer purchasing a DSLR camera might receive different explanations and recommendations based on whether they identify as a beginner or a professional photographer.
This AI expert system could potentially integrate a wide range of product knowledge, including technical specifications, user manuals, expert reviews, and common use cases. It could answer specific questions about product features, compatibility with other items, or even troubleshoot common issues.
If developed and implemented, such a system could potentially improve customer support efficiency and increase sales by providing instant, expert-level advice. It could reduce the workload on human customer service representatives, allowing them to focus on more complex inquiries while ensuring that customers receive immediate, accurate information at any time.
The potential impact of such a system is significant. According to a report by Forrester Research, 53% of customers are likely to abandon their online purchase if they can’t find quick answers to their questions. By providing instant, expert-level advice, e-commerce businesses can potentially improve customer support efficiency and increase sales.
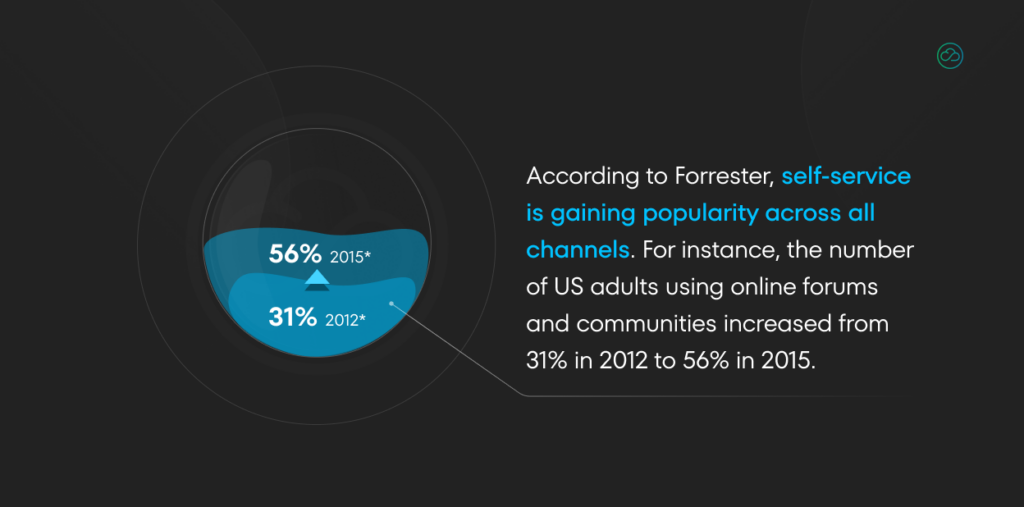
5. Return Reason Analysis
A Return Reason Analyzer leveraging LLM technology could offer a proactive approach to addressing high return rates, a persistent challenge in e-commerce. This system would use natural language processing to analyze free-text return reasons, identifying patterns and insights that might be missed by traditional categorical analysis.
For example, if multiple customers return a piece of clothing citing “fabric feels different than expected,” the system could flag this for the product team to update the description with more accurate fabric details. This level of nuanced understanding would allow businesses to make data-driven improvements to product descriptions, potentially reducing future returns.
The system could categorize return reasons into actionable insights, such as issues with product quality, inaccurate descriptions, sizing problems, or shipping damages. It could then generate reports highlighting the most common issues for each product or product category, allowing businesses to prioritize improvements effectively.
The impact of such a system could be substantial. The National Retail Federation reports that return rates for online purchases average 20.8%. By gaining a more detailed understanding of return reasons, businesses can take targeted actions to reduce this figure, potentially improving profitability and customer satisfaction.
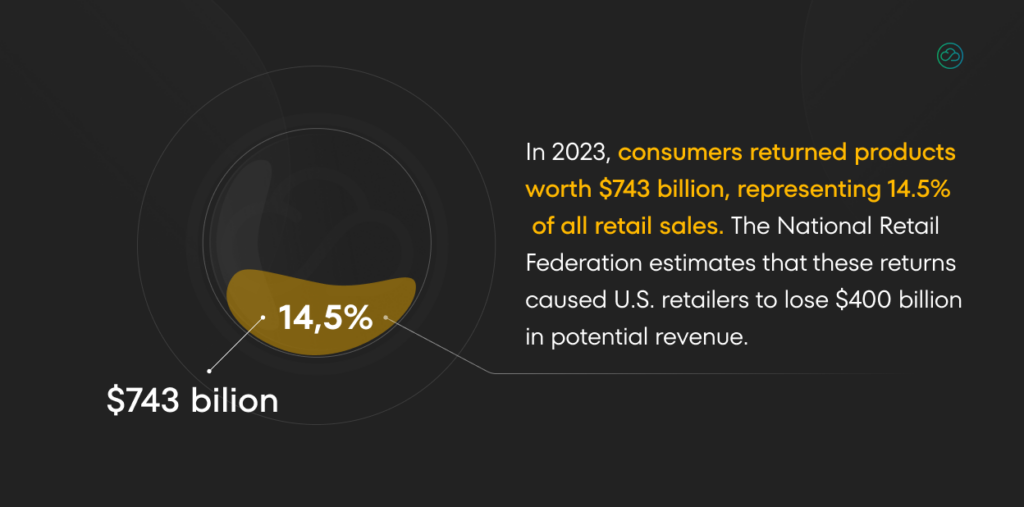
Conclusion
These five concepts for applying LLM technology in e-commerce address critical challenges faced by large and medium-sized online retailers. They represent exciting possibilities for enhancing product discovery, providing personalized expert advice, and leveraging customer feedback more effectively.
Most of these ideas can be implemented as dedicated plugins or modules, making them adaptable to various e-commerce platforms.
Whether you’re using open-source solutions like Magento or WooCommerce, or commercial platforms such as Shopify or Salesforce Commerce Cloud, integrating these LLM-powered tools is feasible. This flexibility ensures that businesses can enhance their existing systems without a complete overhaul of their e-commerce infrastructure.
If you’re interested in implementing LLM-powered solutions in your e-commerce operations, NubiSoft can help.
With our experience in both LLM implementation and e-commerce development, we’re well-positioned to advise on and create tailored solutions for your specific business needs. Contact us to discuss how we can help leverage the power of LLMs to transform your e-commerce operations.