Introduction
With growing internal problems in the healthcare sector – such as, for example, a 90% burnout rate among physicians – it seems a natural step to look to technology for a solution.
The most promising direction in recent years is of course Artificial Intelligence. Properly implemented, AI can relieve doctors of some tasks, allowing them to spend more time with patients or rest, ultimately aiming to improve health outcomes.
But the real question is what do doctors themselves think about artificial intelligence technologies in healthcare?
Two German researchers, Sophie Isabelle Lambert and Murielle Madi, set out to understand the medical community’s evolving relationship with artificial intelligence and to identify the factors that most influence doctors’ acceptance of AI tools.
In a comprehensive review published in the prestigious journal Nature, they analyzed more than 21,000 (!!) publications, ultimately selecting 42 studies that met their rigorous criteria. Based on these, they identified several factors that are most important for the successful implementation of AI in medicine.
Our blog post explores key findings from Lambert and Madi’s work, focusing on three critical questions related to the integration of AI technologies in healthcare organizations:
- How do doctors feel about using AI in their practice?
- What worries do doctors have about AI in medicine?
- What features are doctors hoping to see in AI tools?
Let’s dive in!
How do doctors feel about using AI in their practice?
There isn’t a clear consensus on whether doctors and nurses believe AI will help them do their jobs better.
Some studies suggest that doctors find AI tools helpful. Other studies, however, suggest that in some situations, such tools are more likely to get in the way.
According to a survey conducted by the American Medical Association, 65% of participating physicians see advantages in using artificial intelligence in healthcare.
Specifically, 56% of respondents believe that AI can enhance:
- care coordination,
- patient convenience,
- patient safety.
So, the survey above shows that the majority of physicians are positive about the possibilities offered by artificial intelligence. This is also reflected in doctors’ opinions after pilot implementations:
- Some studies show that physicians believe that clinical decision support systems (CDSS) reduce the rate of medical errors through alerts and recommendations
- In another study, 85% of 100 surgeons, anesthesiologists, and nurses found alerts useful for early detection of complications.
- In a survey of radiology department workers, 51.9% of participants think that AI applications will save time for radiologists.
Research projects focused on the adoption of AI in radiology and the integration of machine learning into clinical operations, medical doctors and nursing staff viewed AI as precise and grounded in ample scientific proof when it came to diagnosis, impartiality, and information quality.
Implementing these systems in emergency rooms (ERs), however, could lead to an increase in errors. A separate study identified usability challenges, with respondents reporting alert fatigue due to the high volume of alerts and a tendency for some physicians to disregard them.
What worries do doctors have about AI in medicine?
In the studies cited, physicians’ concerns about using AI tools relate to 5 areas:
- Reliability,
- Transparency,
- Legal clarity,
- Efficiency in terms of time and workload
- Being replaced by AI
Concerns about the reliability of AI systems
Several studies revealed a recurring theme regarding reliability: concern about the quality of the obtained information.
- In 3 surveys, participants found it insufficient to correctly make a diagnosis, which translated into a general doubt about the accuracy of diagnostic systems.
- In another study, respondents noted that decision support systems can be helpful but have limited capabilities.
- 49.3% of physicians in a survey evaluating the use of artificial intelligence in ophthalmology indicated that the quality of the system is difficult to guarantee.
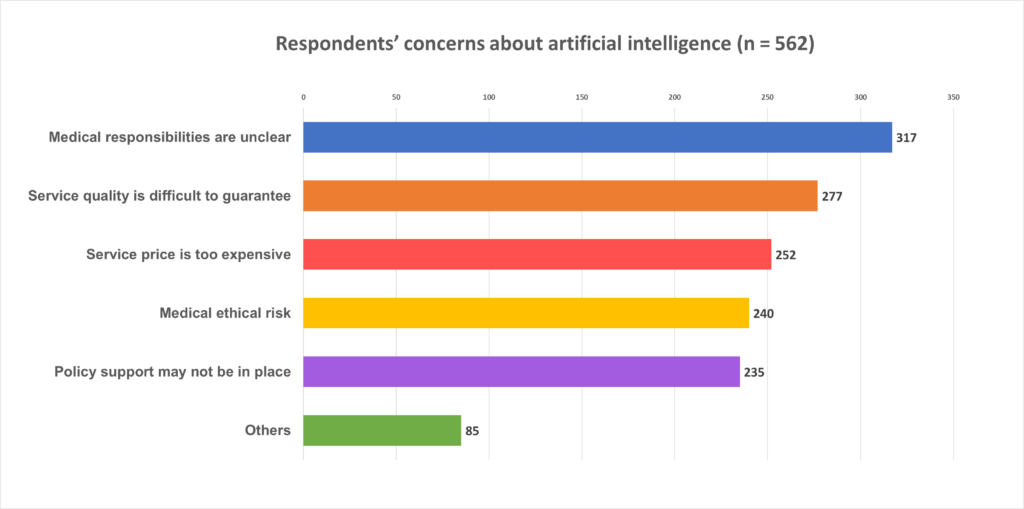
source: https://link.springer.com/article/10.1186/s12913-021-07044-5/tables/7
The belief that AI systems will create more work
The belief that these tools will create additional workloads rather than streamline work also seems to be a barrier to adoption.
- A study investigating the implementation of an AI tool to support neurosurgeons demonstrated that, when asked about barriers hindering the adoption of such a tool, 33% of respondents cited “additional training”. The same proportion of respondents indicated “controversial software reliability” as a barrier
- One study investigating factors affecting the adoption of CDSS systems found that they were perceived as time-consuming due to the need for manual data entry and the steep learning curve associated with new software
- Doctors involved in the robotic surgery study were concerned that some procedures involving artificial intelligence tools might take longer, but nurses and other operating room staff did not share these concerns.
Limited understanding of how the tool works
A study conducted a multidisciplinary analysis of AI transparency in healthcare, examining both legal frameworks and technical perspectives. Researchers reviewed EU legislation, policy documents, and computer science literature to develop a comprehensive view of transparency issues.
Based on this analysis, the main concerns of physicians regarding the transparency and adaptability of AI in healthcare include:
- The ability to understand and explain AI decisions to patients, as required for informed consent.
- The need for tailored, comprehensible explanations about AI functionality and outcomes.
- The challenge of verifying AI system accuracy and safety for specific patients.
These concerns align with another study where participants considering a new predictive machine learning system emphasized the need for clear and well-researched guidelines for its development.
Additionally, a separate study on implementing a CDSS in pediatrics found that unfamiliarity with the system led to resistance from doctors
Lack of legal knowledge about liability in case of system errors
Beyond transparency in system function, physicians also have concerns about who is responsible if the AI fails.
This uncertainty can lead to apprehension and hinder adoption.
However, studies have shown a positive aspect of AI implementation: maintaining patient privacy. Participants in two studies have highlighted the importance of confidentiality, indicating that AI systems should effectively uphold this principle.
Concerns that AI will take over healthcare jobs
A major concern among healthcare professionals is the potential for AI to replace them.
The fear that AI will replace doctors is widespread and varies by specialty and career stage. It’s hard to say for sure that all doctors are afraid of AI.
Based on this comprehensive systematic review of physicians’ and medical students’ acceptance, understanding, and attitudes toward clinical AI, it can be said that most physicians do not significantly fear being completely replaced by AI:
- In the majority of studies (31 out of 40) that addressed this topic, less than half of respondents believed AI could replace human physicians.
- In the authors’ own survey, 68% of respondents disagreed that physicians will be replaced by AI in the future.
It is worth noting, however, that according to some studies, certain medical specialties still have concerns about AI. One example is radiology – in this survey, about 40% of respondents said they are concerned about being replaced by AI in the future. Another research, by Zheng et al., found most ophthalmologists (77%) and technicians (57.9%) believe AI will play a role in their field, while 24% of these professionals believe it will completely replace doctors.
These anxieties can create resistance to adopting AI tools. Addressing these concerns should be a key part of implementing AI in healthcare.
What features are doctors hoping to see in AI tools?
The research highlights several factors that influence the adoption of artificial intelligence solutions. They are:
- Safety
- Intuitiveness of the system
- Integration with workflow
- True help
- Advanced training
Fewer errors increase trust
Diagnostic support systems can have errors, but those powered by artificial intelligence (AI) generally have fewer compared to traditional programs (link). This reduction in errors is particularly pronounced in systems designed for straightforward tasks, leading to a heightened sense of trust in the tool’s capabilities.
Therefore, when designing an AI system, it is safer to create a less complex solution, that is, one that helps with simpler tasks.
Easy-to-use systems have higher adoption rates
The findings of this study suggest that ease of use, integration into existing workflows, and simplicity were important factors in clinicians adopting and valuing the system over time.
The study emphasizes that reducing complexity and making the system compatible with existing practices were key to its successful implementation. According to the survey, AI is generally perceived as easy to use – in this survey, 77% of healthcare professionals said they found AI easy to use.
In the study on perceptions of CDSS, systems that are intuitive, simple, and easy to learn were highly valued by healthcare providers. In the overall study on AI in healthcare, as many as 70% of respondents agreed with every statement about the importance of usability of AI programs.
This also applies to the way they interact with the user, i.e. the doctor. Several studies have observed the phenomenon of alarm fatigue. Too high sensitivity of these indicators can negatively affect the customer satisfaction index. It also leads to security risks. Studies have shown that in critical situations, warnings from the system were simply ignored.
The system should be integrated with the workflow
Integrating the system into the existing workflow is also important. Participants in the study described “required additional tasks” as “undesirable” when using AI systems. The problem is significant because in another study, nearly 40% of anesthesiologists said they could not seamlessly integrate the system into their clinical routine. Radiologists who have used an AI system that lacks automation and consistency with common standards have rated such a system as unreliable (link).
In contrast, if the system demonstrates consistency with common standards and is tailored to the tasks of a medical professional, it is welcomed (link).
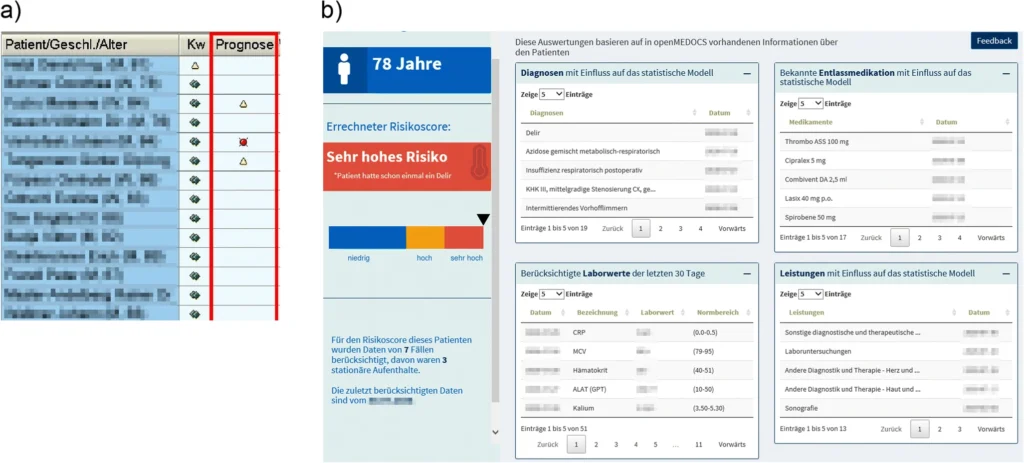
(a) and in a web application presenting patient specific features for prediction
(b). For the sample patient, a very high risk of delirium is predicted (in red)
source: Technology Acceptance of a Machine Learning Algorithm Predicting Delirium in a Clinical Setting: a Mixed-Methods Study
If the system really helps, it is easier to accept.
Research found that nearly 90% of participants expected their workload to increase after the integration of AI technologies. Lack of time, documentation overload, and patient volume are common issues in critical care, so the added burden of dealing with “yet another program” can be a major objection to implementing AI systems.
However, if the system is able to reduce a physician’s workload and realistically help him or her, there is a better chance of acceptance (link)
Training affects acceptability
Also important to the successful implementation of AI in health care is the prior training of staff. In studies of machine learning systems, users without prior experience with such tools felt overwhelmed.
As training increases, so does the percentage of people using AI tools.
-
- One training session: While effective for some, only half of the nurses who received one training session utilized the AI system.
- Two training sessions: A significant improvement was observed with 83% of nurses using the AI system after completing two training sessions.
- Three training sessions: The highest effectiveness was achieved with all nurses (100%) using the AI system after completing three training sessions (link)
Who are we?
At NubiSoft, we develop software for the healthcare industry. We are a trusted technology partner for medical institutions as well as for companies whose end users are medical professionals.
We are certain that well-designed solutions can make a real difference in the work of medical professionals.
We are ready to help your company join the AI revolution safely and sustainably. Contact us when you think you’re ready for it, too.