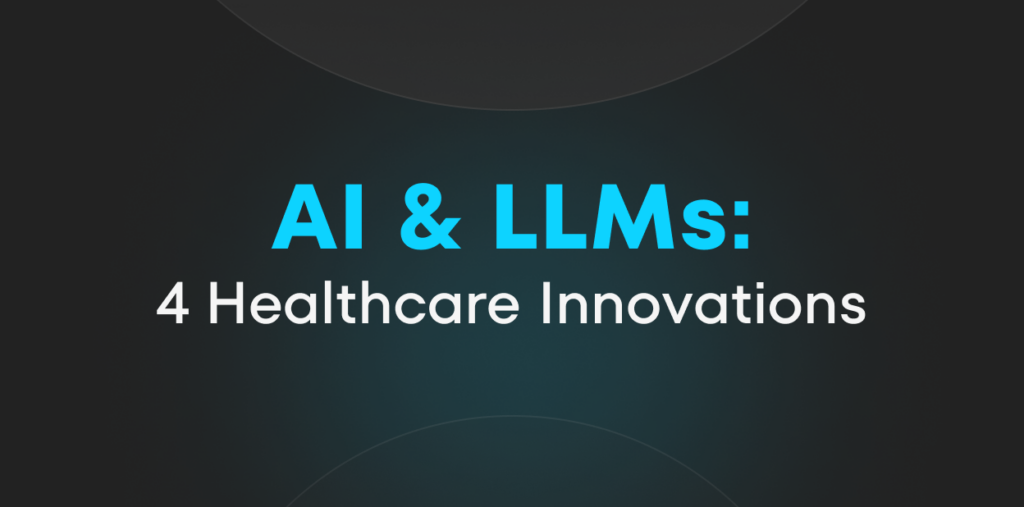
Artificial intelligence in healthcare is no longer a tale of the future – it is a reality that is happening right before our eyes. With more and more tools on the market to help doctors perform tests, make diagnoses, or develop treatment plans, we don’t think anyone doubts that artificial intelligence is a true revolution in healthcare.
By 2030, the medical artificial intelligence market is expected to be worth $188 billion.
According to experts, implementing artificial intelligence in healthcare will enable doctors to increase the time they spend with patients by nearly 20%, thanks to reducing the time spent on administrative tasks. In addition, nurses are expected to spend approximately 8% more time with patients due to a reduction in time spent on administrative and regulatory tasks.
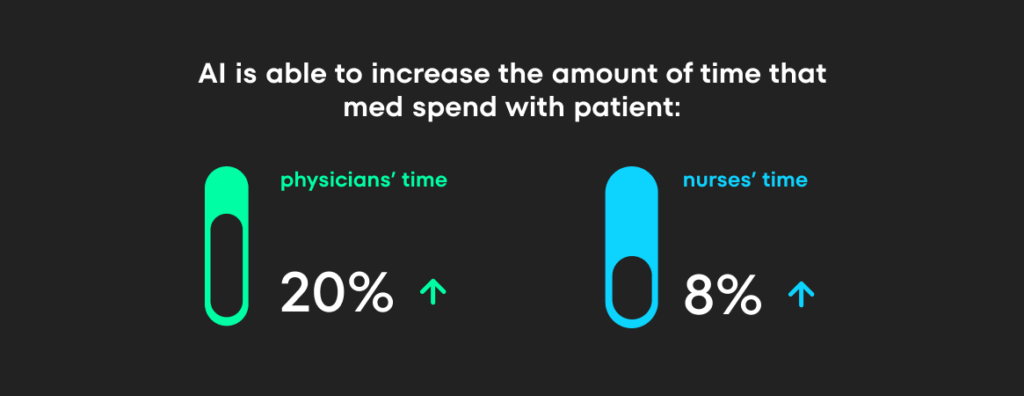
The numbers speak for themselves: AI is having a major moment in healthcare. The hype is real, but so are the misconceptions. Some people think AI will be our healthcare superhero, solving every problem. Others worry it’ll replace doctors entirely.
Not quite! AI has limits, and we need to be clear on those. That’s why we’re here to cut through the noise. This article dives into real-world examples of how large language models (LLMs) are already helping medical professionals.
By understanding how these tools work and what they’re doing today, we can build smarter and more effective solutions for tomorrow.
What is the Large Language Model?
First, let’s explain what an LLM is. A Large Language Model is a type of artificial intelligence algorithm that uses deep learning techniques and large data sets to understand and produce human-like output. They can predict the next word in a sequence based on the previous words. LLMs can answer questions, write essays, summarize text, translate languages, and even write code.
Their capabilities make them applicable to a variety of healthcare areas, 4 of which we have highlighted below and selected representative case studies for each. These areas are:
LLMs in healthcare administration
The first area of healthcare where AI is poised to make a big difference is the administrative side of healthcare.
In 2018 the American Medical Association conducted a study which was a deep dive into the time physicians spent on administrative tasks and paperwork. The report’s authors collected data from more than 20,000 physicians across nearly 30 specialties, and the results were nothing short of “mind-boggling” revealing that:
- Nearly a third of physicians spent 20 hours or more a week on administrative tasks.
- Despite the increase in administrative work, the time physicians spent seeing patients remained relatively unchanged.
Mind Matters (Surrey and Borders Partnership NHS Foundation Trust) faced a similar problem. An increase in referrals and recruitment difficulties led to a significant administrative burden at Mind Matters. This led to inefficiencies in the referral process and negatively impacted staff wellbeing.
In response to these challenges, the Mind Matters team decided to implement an AI chatbot.
The goal was to :
- simplify the referral process,
- reduce administrative confusion,
- increase team efficiency,
- improve staff well-being.
The chatbot was available on the website. It was the patient’s first contact with the facility. It engaged patients in a natural conversation and guided them through the referral process. This replaced long and cumbersome registration forms and made the whole process more flexible (for example, the bot did not ask non-smokers questions about smoking). Based on the answers, there was also a process of initial redirection to more appropriate services if indicated. The system applied the appropriate notification if the patient’s condition required the fastest response.
Results?
- Nearly all patients who provided feedback reported that Limbic was helpful (98,72%)
- 30% increase in referral handling
- Average medical worker time saved is 15 minutes per referral, which translated into 2,000 hours (as of the date of writing the case study)
- Nearly 20% of referrals were identified as ineligible and redirected to a more appropriate service, creating additional capacity for the service.
Limbic was connected to NHS Spine (the national IT infrastructure supporting health and social services in England), so it could independently verify the eligibility of referrals.
Over time, Limbic has become the most popular mental health triage chatbot within the NHS and has been adopted by 17 regions across the UK. Currently accessible to over 9 million people, Limbic has facilitated the release of over 20,000 clinical hours for IAPT services.
Education
The field of medicine is inextricably linked to education. Here, LLMs emerge as transformative tools with the potential to significantly enhance the learning experience.
And to be clear – LLMs are not designed to facilitate shortcuts in medical education.
Rather, they offer the opportunity to equip students with more effective learning methodologies that transcend traditional rote memorization. Imagine a shift from textbook-heavy learning to a dynamic, interactive approach that fosters deeper understanding.
Relevance to the medical sector
This focus on enhanced learning is particularly relevant given the pressing issue of the healthcare workforce shortage. One contributing factor is the notoriously demanding nature of medical education. LLMs, with their ability to work creatively with vast amounts of information, have the potential to democratize medical knowledge, making it more accessible and efficient to learn.
Moreover, the problem is not unique to students.
Medical education is a never-ending process – after all, practicing physicians need to stay abreast of the latest research to improve patient care.
Google MedPaLM and USMLE
Consider the advancements made by Google.
In December 2022, their Med-PaLM model, trained on a comprehensive dataset of medical information, demonstrably achieved success on an exam simulating the USMLE (a crucial licensing exam for doctors in the United States). The model scored an impressive 67.5%, exceeding the passing threshold.
Notably, just a few months later in July 2023, the next generation, Med-PaLM 2, achieved an even higher score of 86.5% on the same test!
How can LLMs support medical education?
It was then realized that properly prepared chatbots could play an important role in the medical education process. Their ability to process large amounts of information, not just text, but also images, charts, and infographics, makes them a powerful tool to assist students in their studies.
Specific ways to support medical education are numerous. One of the most promising ways is to use chatbots as personalized learning assistants that explain certain complex issues in a simpler, more understandable way. Here is an example of such a conversation:
Human: Explain to a five-year-old how the human circulatory system works?
GPT-4: Sure, let’s imagine the human body as a big city. The circulatory system is like the city’s delivery service.
Heart: This is like the delivery center. It’s a strong muscle that pumps blood. It works all the time without any breaks.
Blood: This is like the delivery trucks. They carry oxygen and nutrients (the food and air cells need) to all parts of the body. They also pick up waste, like carbon dioxide, to take it away.
Blood Vessels: These are the roads for the delivery trucks. There are big highways (arteries), smaller streets (capillaries), and return routes (veins). The arteries carry blood away from the heart to the body, capillaries connect arteries to veins in tissues and organs, and veins carry blood back to the heart.
So, the heart sends out the blood trucks on the vessel roads to deliver oxygen and nutrients and pick up waste. Then, they return to the heart to get ready for the next trip. This happens over and over, all day and all night, to keep our body city running smoothly!
Such an assistant can also help creatively develop certain material so that it is easier to remember. Here is an example of using GPT to create a mnemonic.
Prompt: “create a mnemonic for the treatment of pre-eclampsia”
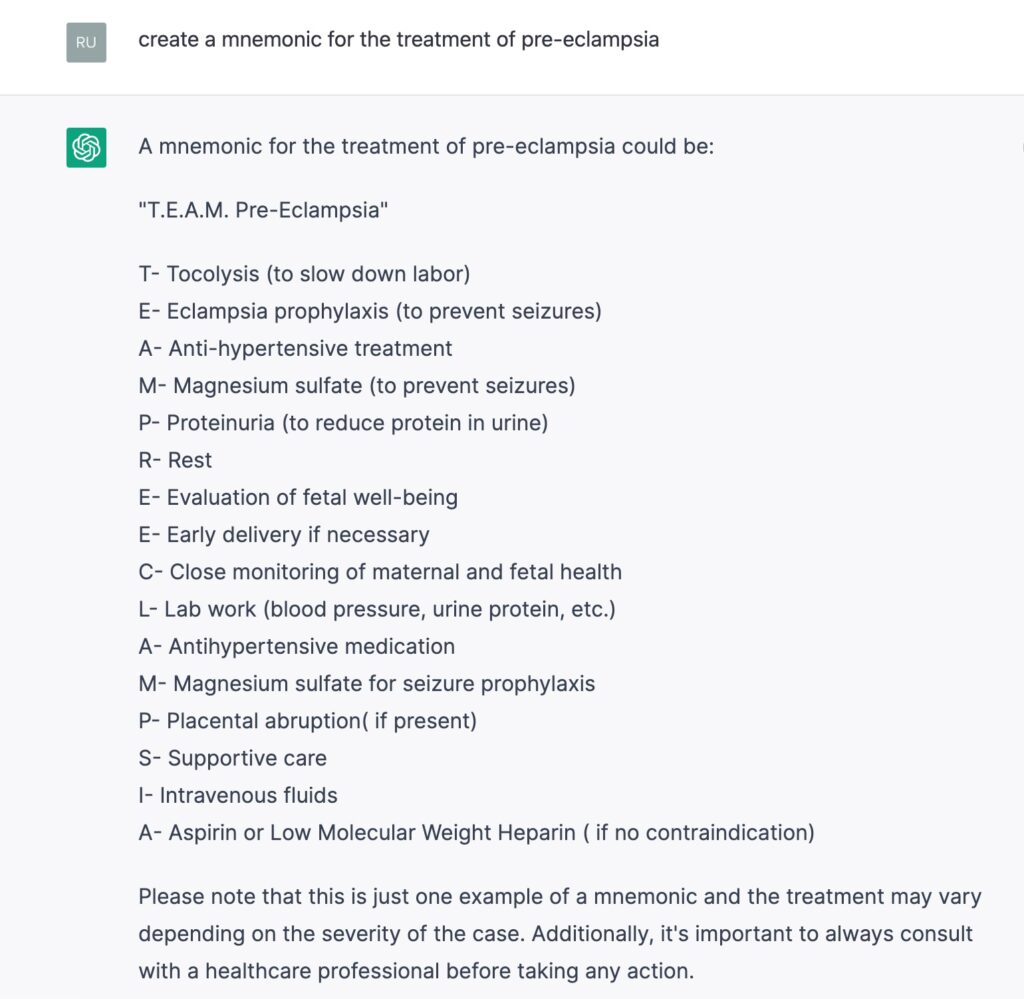
What are the limitations of the LLM in medical education?
While large language models (LLMs) offer immense potential for tool design, it’s important to recognize their limitations. In the above examples, freely available tools such as GPT-3.5 have been used. This has two major drawbacks:
- outdated information
- tendency to generate inaccurate or misleading content (hallucination).
While paid solutions can mitigate these problems, they can’t eliminate them. Therefore, a clear understanding of these limitations is essential when designing tools or crafting prompts.
Below is an example of the potential pitfalls of LLMs in education. While the errors in the LLM’s response may seem apparent, that’s not always the case. Students who lack the skills to effectively verify the information presented by these tools are more susceptible to being misled.
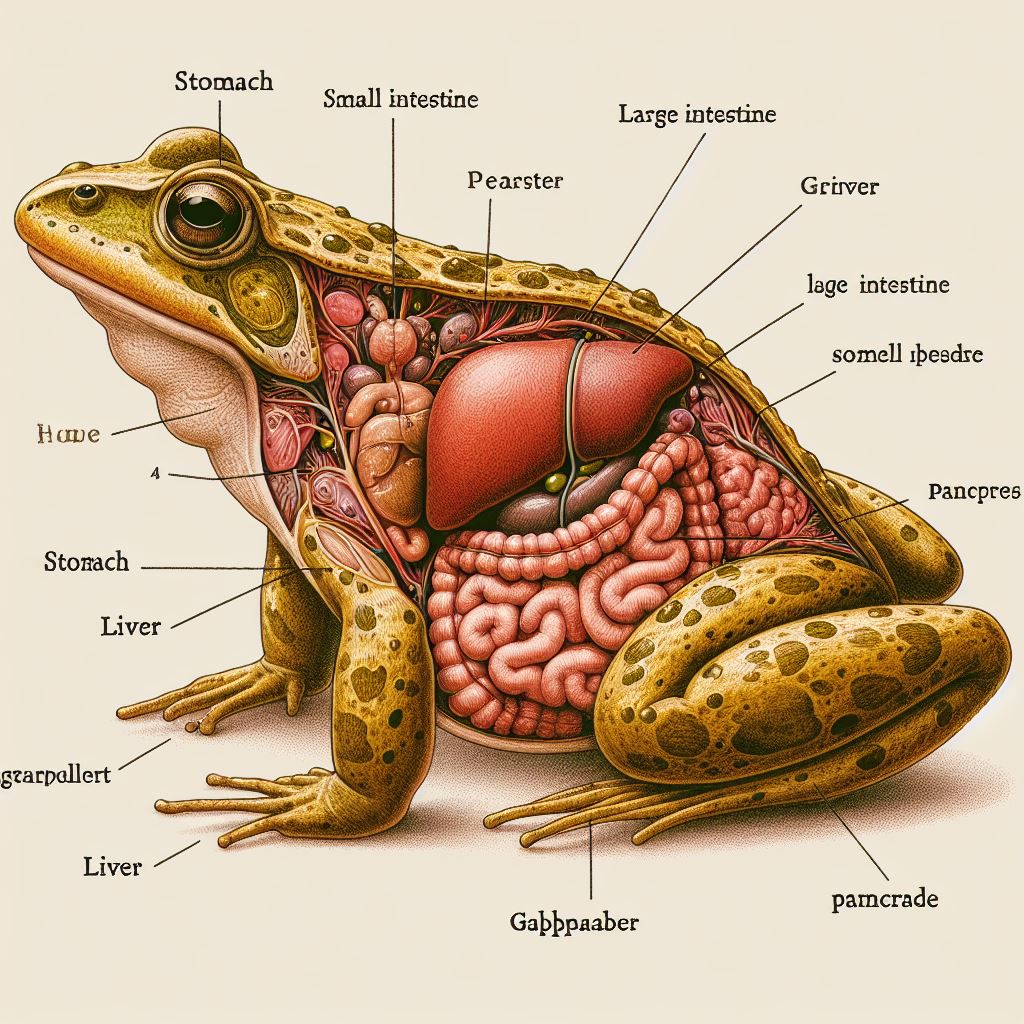
Prompt: “Create an infographic showing how the frog’s digestive system functions.”
How do LLMs improve medical research processes?
Another area of modern medicine where LLMs are already being used is in research. And just as in the case of education, concurrent use cases for tools with this technology in the research process are numerous.
- Data analysis: LLMs can assist in analyzing complex medical data, identifying patterns, and generating insights that can guide research.
- Literature summarization: LLMs can wade through massive amounts of medical research papers and clinical data to identify trends, synthesize information, and generate concise summaries of complex topics.
- Hypothesis generation: LLMs can analyze existing research and patient data to identify potential connections and suggest new hypotheses for further investigation.
- Drug discovery and development: LLMs can be used to analyze vast datasets of molecular structures and biological pathways to help identify potential drug targets and accelerate the development of new treatments.
- Paper Writing: LLMs can assist in writing research papers, ensuring they are well-structured and adhere to the relevant style guides.
LLMs in the drug discovery process
The discovery of new drugs is a complex, time-consuming, and costly process. It often involves years of meticulous research, rigorous testing, and substantial financial investment.
The traditional drug discovery process can take up to 15 years and cost over $2.6 billion, according to a study by the Tufts Center for the Study of Drug Development.
In this context, LLMs have emerged as a transformative tool in the medical research field, particularly in drug discovery. Its capabilities allow it to analyze vast amounts of the biomedical literature, patents, and clinical trial data in a fraction of the time it would take a human researcher. This can significantly speed up the initial stages of drug discovery, which involve identifying potential drug targets and candidate molecules.
BenevolentAI case study
A positive example of the use of such technology is BenevolentAI, a company based in the UK. They used an LLM they developed to identify a potential treatment for COVID-19. The company’s AI-based hypothesis was that “baricitinib”, a drug approved for the treatment of rheumatoid arthritis and other diseases, might be effective in treating COVID-19.
This hypothesis was validated through a large-scale randomized clinical trial sponsored by the National Institute of Allergy and Infectious Diseases (NIAID). The trial included more than 1,000 patients and began on May 8 to assess the efficacy and safety of “baricitinib” plus remdesivir versus remdesivir in hospitalized patients with COVID-19.
The results showed that “baricitinib” in combination with remdesivir achieved the primary endpoint of the study, which was a statistically significant reduction in the time to recovery for patients in comparison with remdesivir alone.
Furthermore, data from Eli Lilly’s COV-BARRIER trial showed that “baricitinib” reduced deaths in hospitalized COVID-19 patients by 38%. This is the largest clinical effect reported to date for a reduction in mortality in this patient population.
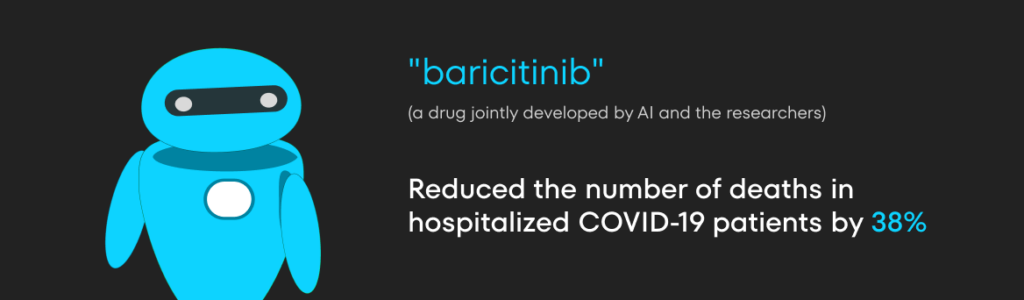
In summary, the implementation of this solution has yielded promising results. The use of AI by BenevolentAI in research for COVID-19 treatment led to the identification of a potential treatment, which was validated through clinical trials and showed promising results in reducing recovery time and mortality in hospitalized COVID-19 patients. The LLMs suggest novel biological targets that could evolve into a drug discovery program if experimentally validated, deemed safe, and able to provide an opportunity to differentiate.
However, building trust in the methodology and preventing algorithmic biases remain key barriers to AI deployment.
How LLMs help diagnose?
Many people might think using AI in medicine is all about diagnosing illnesses. We can easily imagine a scenario where a chatbot asks a patient a few questions, analyzes their answers along with other information like treatment history, and then – poof! – the AI delivers a diagnosis and treatment plan.
But the reality is far more nuanced. Diagnosing a patient is a complex puzzle that requires a doctor’s knowledge, in-depth data analysis, and even strong interpersonal skills to understand the patient’s experience.
Recreating that process entirely with a tool is extremely difficult, if not impossible, at this stage.
It is easier, although still a significant challenge, to create an AI tool that supports the work of a human, thus saving his time and energy. Based on real-life examples, LLMs can:
- improve clinical workflow efficiency,
- reduce diagnostic errors,
- assist healthcare professionals in making timely and accurate diagnoses.
Can LLMs assist with Differential Diagnosis?
A real-life example of the use of LLMs in diagnostics is the test application of such a tool to support differential diagnosis. The study was conducted, again, by Google. They used a model with a transformative architecture – PaLM 2, fine-tuned with data from the medical domain.
The objective was to introduce an LLM optimized for diagnostic reasoning and evaluate its ability to generate a DDx alone or as an aid to clinicians. DDX is a process where a doctor considers multiple possible conditions that could be causing a patient’s symptoms.
The study involved 20 clinicians who evaluated 302 challenging, real-world medical cases from the New England Journal of Medicine (NEJM) case reports. Each case report was read by two clinicians who were randomized to one of two support conditions:
- From search engines and standard medical resources,
- or LLM support in addition to these tools.
All clinicians performed a baseline, unassisted DDx before using each of the assistive tools.
The results were promising.
The LLM tool was very good at suggesting possible diagnoses (differential diagnoses). In fact, it did better than doctors on its own!
- Almost 60% accuracy in the top 10 diagnoses (vs. only 33% for doctors).
- Doctors who used the LLM to help them also came up with better diagnoses than doctors who didn’t.
In conclusion, this study suggests that LLMs hold promise for improving clinicians’ diagnostic reasoning and accuracy, especially in complex cases.
Further real-world evaluation is warranted to assess their ability to empower physicians and expand patient access to specialist-level expertise.
Summary
The AI-powered tech revolution is just starting, and its reach is expanding across industries. However, healthcare presents a unique challenge. Here, the stakes couldn’t be higher, leading to a web of regulations and a natural conservatism when it comes to adopting new ideas.
This means some promising solutions are stuck in testing, delaying their real-world impact.
Despite this hurdle, these advancements offer a glimpse into the future of healthcare, hinting at where the market is headed. The companies that can thoughtfully and swiftly implement these solutions are the ones to big win.
Who we are?
At NubiSoft, we specialize in working with the healthcare sector on dedicated solutions using AI.
We believe that properly designed solutions can make a real difference in the work of medical professionals.
We are ready to help your company join the AI revolution safely and sustainably. Contact us when you think you’re ready for it too.
Sources:
- https://www.statista.com/statistics/1334826/ai-in-healthcare-market-size-worldwide/
- https://radixweb.com/blog/ai-in-healthcare-statistics
- https://www.ama-assn.org/practice-management/sustainability/do-you-spend-more-time-administrative-tasks-your-peers
- https://www.statnews.com/2023/02/01/promises-pitfalls-chatgpt-assisted-medicine/
- https://limbic.ai/nhs-talking-therapies
- https://transform.england.nhs.uk/key-tools-and-info/digital-playbooks/workforce-digital-playbook/using-an-ai-chatbot-to-streamline-mental-health-referrals/
- https://sites.research.google/med-palm/
- https://mededu.jmir.org/2023/1/e46885
- https://www.ncbi.nlm.nih.gov/pmc/articles/PMC10463084/
- https://lifesciences.n-side.com/blog/what-is-the-average-time-to-bring-a-drug-to-market-in-2022
- https://www.techuk.org/resource/aiweek2023-benevolentai-mon.html
- https://www.prnewswire.com/news-releases/benevolentai-data-from-eli-lillys-cov-barrier-trial-shows-baricitinib-reduced-deaths-in-hospitalised-covid-19-patients-by-38-301265356.html
- https://www.mobihealthnews.com/news/study-google-reveals-llm-helps-accurately-diagnosis-complex-cases